A hybrid Feature Selection Method Based on Binary PSO Algorithm for Microarray Data Classification
Keywords:
Feature selection, Particle Swarm Optimiza- tion algorithm, Wrapper method, Dimension reduction, Multi attribute decision makingAbstract
Microarray technology produces data with a large number of dimensions. The abundance of dimensions in the data makes it challenging for machine learning algorithms to extract meaningful information from it. To overcome this limitation, feature selection (FS) techniques can be applied to reduce the dimensionality of the data. FS is a crucial preprocessing step that allows for the handling of high-dimensional data and facilitates more effective and efficient processing. The primary objective of this paper is to develop an efficient FS method that can effectively reduce the dimensionality of microarray data. The Technique for Order Preference by Similarity to Ideal Solution (TOPSIS) is utilized in the first filtering stage to extract informative features. The best feature subset is then determined by using a Particle Swarm Optimization (PSO) algorithm as a wrapper feature selector to identify ideal features. The proposed approach is evaluated using microarray datasets from the Alzheimer’s Disease Neuroimaging Initiative (ADNI) and compared with other algorithms. The experimental findings indicate that the proposed approach outperforms the benchmark methods in terms of classification accuracy.
Downloads
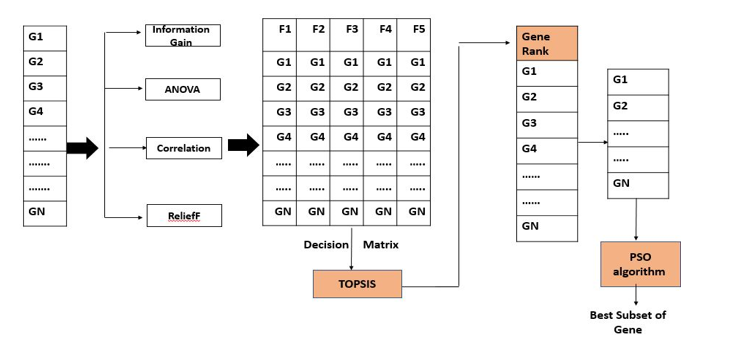
Published
How to Cite
Issue
Section
Copyright (c) 2024 Heba Al.Marwai, Ghaleb H AL-Gaphari

This work is licensed under a Creative Commons Attribution-NonCommercial-NoDerivatives 4.0 International License.